Identifying Patients Using Data Analytics
Use of Machine Learning to Identify Gastroparesis Patients Suitable for Nasal Spray Metoclopramide
Utilizing supervised and unsupervised learning, with hierarchical
patient embeddings to build predictive models.
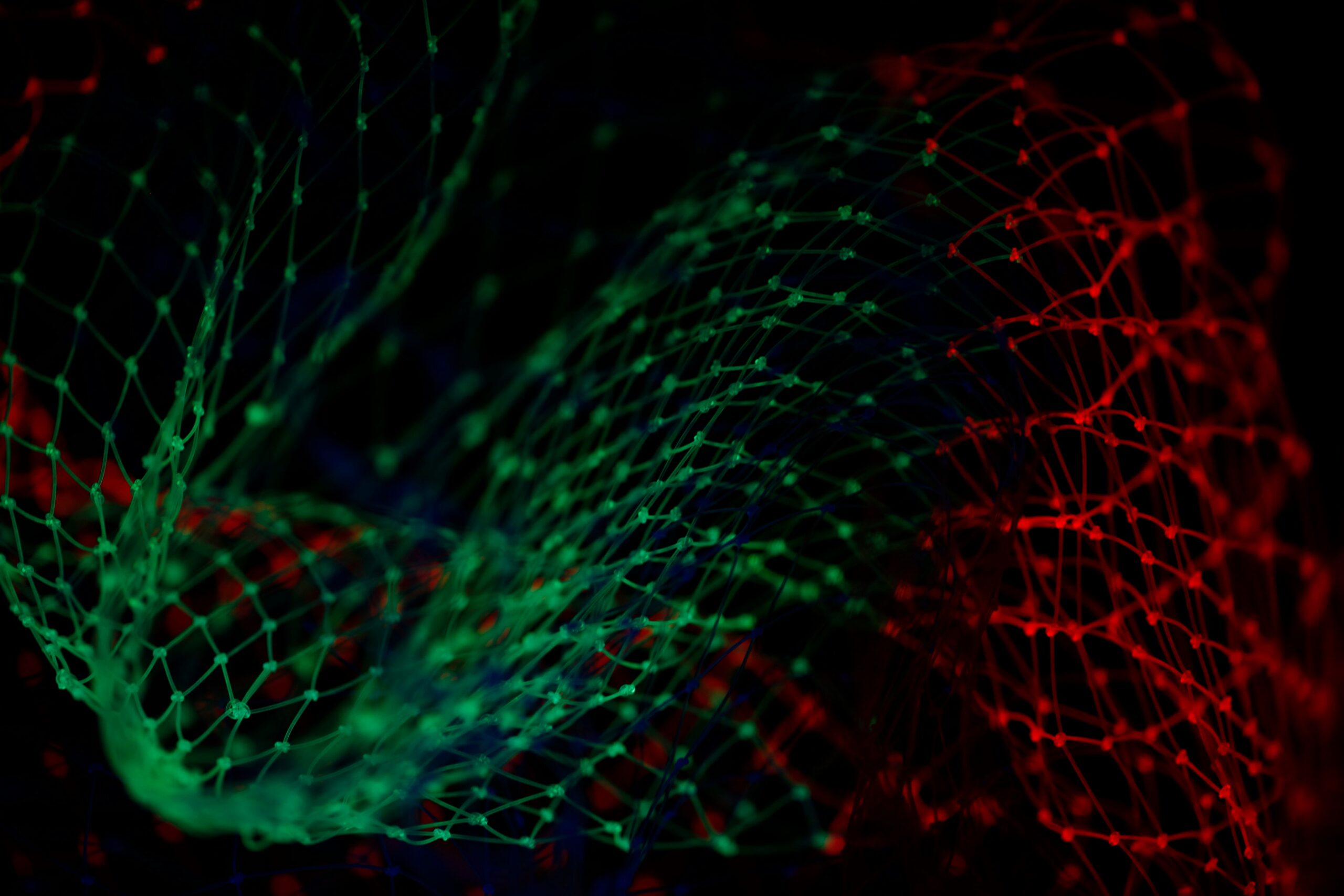
THE CHALLENGE
Oral metoclopramide (OMCP) is the first-line treatment for gastroparesis (GP), but nausea and vomiting may reduce OMCP tolerability, and diabetes may delay oral absorption. In 2020, the FDA approved a nasal spray formulation of metoclopramide (NMCP). However, the clinical profile of patients who could benefit from NMCP in clinical practice is not well established, in part because there is increasing evidence that GP and functional dyspepsia (FD) may be on a spectrum of gastric dysfunction and because patients with clinical phenotypes meeting the criteria for the Gastroparesis Cardinal Symptom Index (GCSI) are not always identified with the clinical or administrative diagnosis of GP in electronic health records and claims databases, respectively.
A midsize pharmaceutical company needed to identify patients with a similar clinical profile who might benefit from NMCP. EVERSANA was contracted to examine the clinical profiles of current patients with GP on NMCP using administrative claims data, then develop a predictive model to identify these patients.
THE SOLUTION
The project faced several challenges, including the complexity of GP and its lack of consistent identification in administrative claims. Additionally, compiling comprehensive and accurate patient data posed significant hurdles, requiring meticulous validation and integration of information from various sources. The wide variety of potential factors that can influence a patient’s treatment type requires intensive feature engineering and statistical techniques.
A retrospective review of 271 patients who had a prescription for NMCP was conducted on EVERSANA’s open claims database (Cohort 1). Using mutual information (MI), unique claims features of Cohort 1 (ICD-10, HCPCS codes and prescriptions) were compared with those of 998 control patients (Cohort 2). The most significant differentiating features between the two cohorts, and prevalent in NMCP patients, were used as input into a gradient-boosting classifier trained to predict the initiation of NMCP.
The model was used to identify patients in the database with a clinical journey profile similar to Cohort 1, but who had not yet received NMCP.
Then, unsupervised learning was used to cluster hierarchical embeddings of the patient journeys, which identified Mild, Moderate and Severe segments of patients. Additional predictive models were designed to sort potential patients into these categories.
THE RESULTS
The designed predictive models identified nearly 1.8 million additional patients with similar clinical profiles as the NMCP patients, utilizing clinical features such as GI dysmotility diagnoses and cumulative metoclopramide doses over time. The patient personas, identified via unsupervised learning algorithms, align well with the clinical measures of mild, moderate and severe comorbidities.
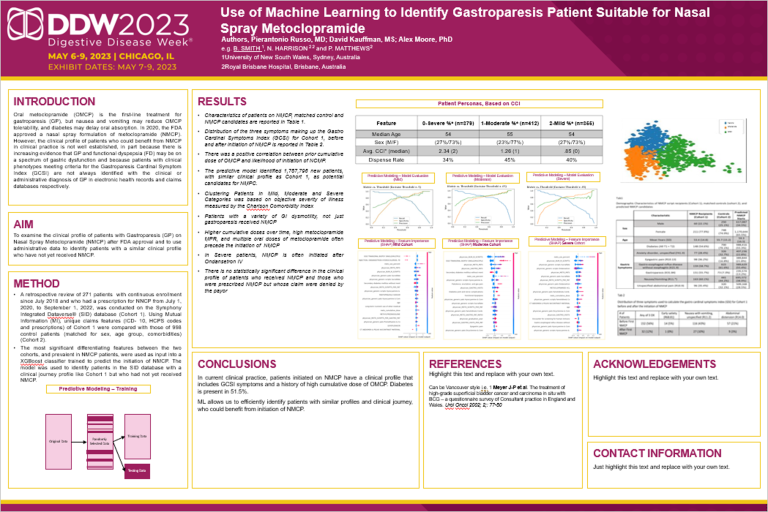
Poster presented at Digestive Disease Week 2023 outlining model performance, feature importance and patient clustering in gastroparesis patients suitable for nasal spray metoclopramide.
Contact Us
WHAT CHALLENGE SHALL WE TAKE ON TOGETHER?
Schedule a meeting with an EVERSANA INTOUCH
Data and Analytics expert.